AWS Lake Formation: Accelerating Data Lake Adoption
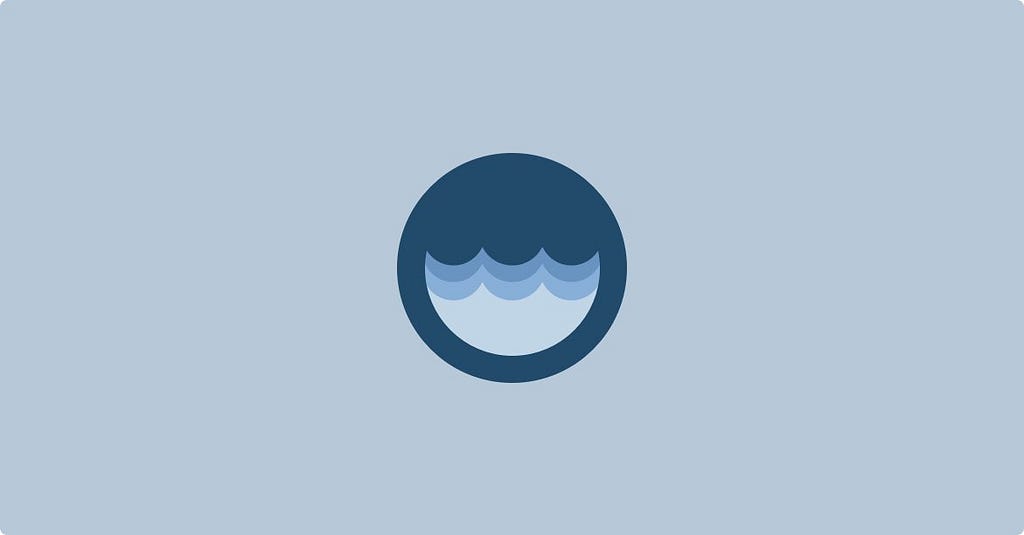
If you read about data lakes, you will often come across a post, guide, documentation, or tweet that will describe setting up a data lake as creating an AWS S3 bucket, some paths, and then dropping files in. VoilĂ , you have a data lake! Not really.
Does a data lake have to be complex to set up? No! However, with a lake formation process employed by AWS, GCP, and Azure you can get up and running more quickly.
In this post, we are focused on the AWS cloud, though many concepts will transcend an AWS deployment to GCP or Azure.
Data Lake vs. Data Warehouse vs. Data Mart
Before we jump into lake formation, we need to baseline what, precisely, we are forming. As we have detailed in a prior post, there are numerous misconceptions and myths about data lakes.
This is how Pentaho co-founder and CTO, James Dixon who coined the term data lake, frames it;
This situation is similar to the way that old school business intelligence and analytic applications were built. End users listed out the questions they want to ask of the data, the attributes necessary to answer those questions were skimmed from the data stream, and bulk loaded into a data mart.
This method works fine until you have a new question to ask. The Data Lake approach solves this problem. You store all of the data in a lake, populate data marts and your data warehouse to satisfy traditional needs, and enable ad-hoc query and reporting on the raw data in the lake for new questions.
The beauty and elegance of a lake should be in its simplicity, agility, and flexibility. Unfortunately, there are several data lake vendors, or data lake solution providers, that are promoting complex, heavy-handed architectures. Heavy-handed architectures represent one of the greatest threats to an efficient, lean, productive data lake.
Overdesigned, Heavy-handed “Closed” Data Lakes
A typical vendor pattern is to wrap, or create, an embedded data lake.
In our example below “System X” reflects a vendor solution that creates a close ecosystem lake. System X forces any data ingress or egress access to the lake to be tightly coupled to the requirements set by vendor System X, not data lake best practices;
An embedded, closed data lake model reflects a data lake in name only. By design this vendor-driven architecture promotes lock-in.
A closed model will limit access by analytic, SQL, ETL, and other tools. Access is governed by proprietary or esoteric drivers that create compatibility nightmares for any tools not blessed by the vendor.
Technical Debt, Big Price Tags
In addition to considerable technical debt, solutions like this come with even bigger price tags. Specialized training, expensive support contracts, avoidance of open-standards…all contribute to a challenging data lake environment for people to work.
A lake becomes a swamp because of the crushing weight of ancillary people, processes, and technologies that are placed in and around it.
As an architecture and operational model, this is vendor lock-in approach is the antithesis of a data lake. You ensure your data lake will not be responsive to change and overly rigid in who and how data is consumed.
As your team gets fed up with this model, they demand you to start looking at alternatives. Sound familiar?
Let’s look at some guiding principles to help you avoid vendors layering an oppressive ecosystem of tools and systems on data lakes.
Open Data Lake Access
Systems X should not be the manifestation of the lake itself. Rather than encapsulate the lake, System X should be a consumer or contributor to the lake
A healthy lake ecosystem is one where you follow a well-abstracted architecture. Do doing ensures you are minimizing the risk of artificially limiting the opportunity lakes represent as System X originally did.
A well-abstracted, open lake ensures sophisticated modeling, data transformation, or wrangling jobs are best-left those that need to consume data, with whatever tools they deem appropriate.
AWS Lake Formation
In an effort to establish a base pattern for a lake, drive consistency, and velocity, AWS established a formalized lake formation process. If you are a GCP or Azure customer, both have similar concepts for lake formation (though the process & tools vary).
Amazon says its lake formation is “a service that allows you to build a secure data lake in days.” In reality, a shell of the AWS lake may only take a few hours to complete.
The power of the AWS Lake formation service is that it organizes a diverse collection of disconnected AWS services it an orchestrated configuration service.
As a result, AWS lake formation is primarily focused on the orchestration of underlying AWS services into a cohesive interface and API. This provides teams with a foundational view of what may be needed for an AWS data lake.
Why did AWS go down this path? Typically, the setup of your AWS data lake will involve IAM, S3, SNS, SQS, Glue, and a host of other AWS services. In the past, you had to set up these services individually. If you were an AWS DevOps person, after some trial and error you likely had most of this automated via Terraform or Ansible. However, like most AWS services, Amazon decided to wrap a user interface to orchestrate its services to increase the time to value.
One important note is that while Amazon will pre-configure its services, it does not mean you have a productive data lake. A productive data lake requires an active data ingestion pipeline or pipelines AND consumers of the contents of the lake. More on this later.
AWS lake formation templates
The AWS data lake formation architecture executes a collection of templates that pre-select an array of AWS services, stitches them together quickly, saving you the hassle of doing each separately.
An AWS lake formation blueprint takes the guesswork out of how to set up a lake within AWS that is self-documenting. While these are preconfigured templates created by AWS, you can certainly modify them for your own purposes. These may act as starting points for refinement.
AWS lake formation pricing
For AWS lake formation pricing, there is technically no charge to run the process. However, you are charged for all the associated AWS services the formation script initializes and starts.
AWS lake formation gaps
Data ingestion to a data lake is an essential consideration for the lake formation process. This includes any data pipeline work needed for loading data from various sources. This provides for the curation of data, partitioning, optimizations, alerts, and authorizations.
Openbridge Data Lake service
If AWS Lake formation is a collection of ingredients, the Openbridge Data Lake service is the cake.
Our service offers a centralized data catalog that not only describes available data sets; it actively manages changes and relationships for ingested data. This creates an “analytics ready” environment allowing teams to employ their choice of analytics, ETL, visualization, and machine learning services.
The Openbridge service will store, catalog, and clean your data faster for your Amazon S3 data lake. Here are just a few of the critical features of the service;
- Automatic partitioning of data — Allows you to optimize the amount of data scanned by each query, thus improving performance and reducing the cost for data stored in s3 as you run queries.
- Automatic conversion to Apache Parquet — Converts data into an efficient and optimized open-source columnar format, Apache Parquet, for use within Azure Data Lake, AWS Athena, or Redshift Spectrum. This lowers costs when you execute queries as the Parquet files are highly optimized for interactive query services like Athena and Spectrum.
- Automatic data compression — With data in Apache Parquet, compression is performed column by column using Snappy, which means not only supports query optimizations, it reduces the size of the data stored in your Amazon S3 bucket or Azure storage system, which reduces costs.
- Automated data catalog and metadata — Ingested data curated, analyzed, and the system “trained” to infer schemas to automate the creation of the database and tables for data that is pushed into the data lake.
- No coding required — Using the Openbridge interface, users can create and configure a data lake. This system is then used as a destination for loading data from different data sources.
- Cleaning — built-in processes to deduplicate to increase data quality.
- Code-free data pipelines — SFTP, AWS Kinesis, and many other supported data sources.
Get Started
If you are looking to build a data lake with Azure Data Lake, Amazon Athena, or Redshift Spectrum, the Openbridge lake formation can get you up and running in a few minutes.
It has never been easier to take advantage of a data lake with a serverless query service like Azure Data Lake Storage, Amazon Athena, and Redshift Spectrum. Our service optimizes and automates the configuration, processing, and loading of data to AWS Athena and Redshift Spectrum, unlocking how users can return query results. With our zero administration, data lake service, you push data from supported data sources, and our service automatically loads it into your data lake for AWS Athena and Redshift Spectrum to query.
References
- See the AWS lake formation documentation
- See the AWS lake formation tutorial
AWS Lake Formation: Accelerating Data Lake Adoption was originally published in Openbridge on Medium, where people are continuing the conversation by highlighting and responding to this story.
source https://blog.openbridge.com/aws-lake-formation-accelerating-data-lake-adoption-d0bf19f99d0a?source=rss----4c5221789b3---4